To Change the World, Set a Bold Target: Moore's Law as Self-Fulfilling Prophecy
Manage episode 446990662 series 3515640
I get a little thrill every time Casey Handmer says that the cost of solar power is going to come down to a penny per kWh (current electricity prices are more like a dime1.)
I love stories about future technology, but I don’t really get excited until someone throws out an aggressive prediction and puts a number on it. With apologies to George Bernard Shaw:
The reasonable forecast adapts itself to the world; the aggressive forecast persists in trying to adapt the world to itself. Therefore all progress depends on the aggressive forecast.
This isn’t just rhetoric. An aggressive forecast really can adapt the world to itself.
A Hard Target Is Inspiring
“If you want to make this presentation more exciting, put some numbers in it”, said no one ever. But a concrete target is more exciting than a vague idea like “electricity is going to get really cheap”.
A number makes things concrete. It makes the vision feel more real, and it allows you to work out the implications. The quantitative predictions offered by Moore’s Law enabled generations of product designers to make the business case for ambitious new gadgets. Inspiring applications can help motivate the effort to make a technical vision come true.
The cost of solar panels has fallen by a factor of 500 (!) over the last half century. We are now entering territory where further reductions – which are more or less guaranteed at this point2 – will become literally world-changing. Casey has been cataloging jaw-dropping potential applications of penny-per-kWh solar power, ranging from using desalination to restore the Salton Sea to restoring Nevada to the lush climate it enjoyed 10,000 years ago.
A numeric target is also terrific for motivating the team that is turning the vision into a reality. At my last startup (Scalyr), we set out to redesign our system to handle more data. We set a goal of raising our data processing capacity, in terabytes per day, from 5 to 150. The “race to 150” became the rallying cry for the entire company, and every successful new test (10 terabytes! 25! 100!) was an occasion for celebration.
A Hard Target Shows the Path
As a technology matures, the industry can settle into a comfortable rut. A hard target – hard both in the sense of “firm” and “difficult” – can give a scientist, engineer, or entrepreneur the courage to rethink a problem from first principles.
For instance, to eliminate greenhouse gas emissions, we will need to produce a lot of clean hydrogen3. The US Department of Energy has set an aggressive target of $1 per kilogram. The US market is currently dominated by a technology known as PEM electrolyzers, which are the most efficient way of using electricity to produce hydrogen. However, they require expensive metals such as iridium and platinum. Startups like Hgen and Casey’s Terraform Industries4 have done the math and determined that PEMs are a dead end – the material requirements mean that PEMs can never reach the $1 cost target.
Instead, Hgen and Terraform are developing alkaline electrolyzers, an alternative technology that had been rejected by previous US manufacturers because it is less efficient. They are betting that with solar power becoming so cheap, the disadvantages of alkaline electrolyzers5 can be overcome. Given the choice between a difficult challenge (improving alkaline electrolyzers) and a mathematical impossibility (hitting the cost target with PEM electrolyzers), and spurred by the prospect of penny-a-kWh solar power and a huge market for $1-per-kg hydrogen, they’ve chosen to make the bold move to alkaline electrolyzers6.
At Scalyr (my startup again), we also used numeric targets to help make strategic decisions. At one point, we were struggling financially because the large data volumes we were processing led to a huge server bill. I did some back-of-the-envelope calculations to estimate the maximum possible efficiency for the type of system we operated. To my astonishment, the theoretical limit was roughly 1000 times lower than what we were spending. This led to us betting the company that we could slash costs, and taking radical steps to get there. Seven years later, Scalyr has reduced spending per terabyte by a factor of 54 – roughly a 77% efficiency increase per year.
At Scalyr, an aggressive target spurred us to find ways of eliminating entire subsystems from our data processing pipeline. The same principle led SpaceX to develop reusable rockets, Terraform Industries to design an alkaline electrolyzer that can be connected directly to a solar panel7, and Apple to design a phone that didn’t need a keypad.
If You Forecast It, They Will Come
Moore’s Law, of course, is the famous observation that the number of transistors in a chip doubles every two years. This trend held fast for an astonishing half century, before finally slowing down in the 2010s. From 1970 to 2010, transistor counts increased by a factor of 1,000,000! These statistics get quoted all the time, but they never get less astonishing.
Progress in integrated circuits began before Gordon Moore originally articulated what became known as Moore’s Law. He was observing an existing trend. However, as that trend continued year after year, it came to be viewed as an actual law, and that perception in turn supported the investments needed for the trend to continue. It became a self-fulfilling prophecy. Huge investments in technologies like extreme ultraviolet lithography and “atomic-level sandpaper8” have supported Moore’s Law… but it was belief in that very law which motivated those investments in the first place. I asked Claude to explain further:
Companies like Intel, IBM, and AMD used Moore's prediction as a roadmap, setting their research and development timelines to meet the expected doubling of transistor counts every two years. This created a powerful feedback loop: businesses invested heavily in hitting these targets because their competitors were doing the same, while customers came to expect regular performance improvements on this timeline. The semiconductor industry even coordinated its planning through the International Technology Roadmap for Semiconductors (ITRS), which used Moore's Law as a baseline for setting industry-wide goals. Market expectations and product cycles became synchronized to this rhythm, with software companies developing more demanding applications in anticipation of faster hardware, and hardware companies racing to meet these demands. The predictability of Moore's Law also gave investors confidence to fund the increasingly expensive semiconductor fabrication plants needed to maintain this pace, turning what started as an empirical observation into a self-sustaining cycle of innovation and investment.
It’s not unusual for a forecast to become self-fulfilling. The relentless decrease in battery prices has created an air of inevitability around the transition to electric vehicles, spurring investments which support further price decreases. Models show that to halt global warming, we will need ways of removing CO₂ from the atmosphere at a price no higher than $100 per ton, and hundreds of startups are now working to do just that. Once people start to believe that a technology can reach a certain price point, suppliers will work to accomplish it (spurred by the fear that a competitor will get there first), and customers who can take advantage of it will arise to create demand.
Let’s Set More Hard Targets for Progress
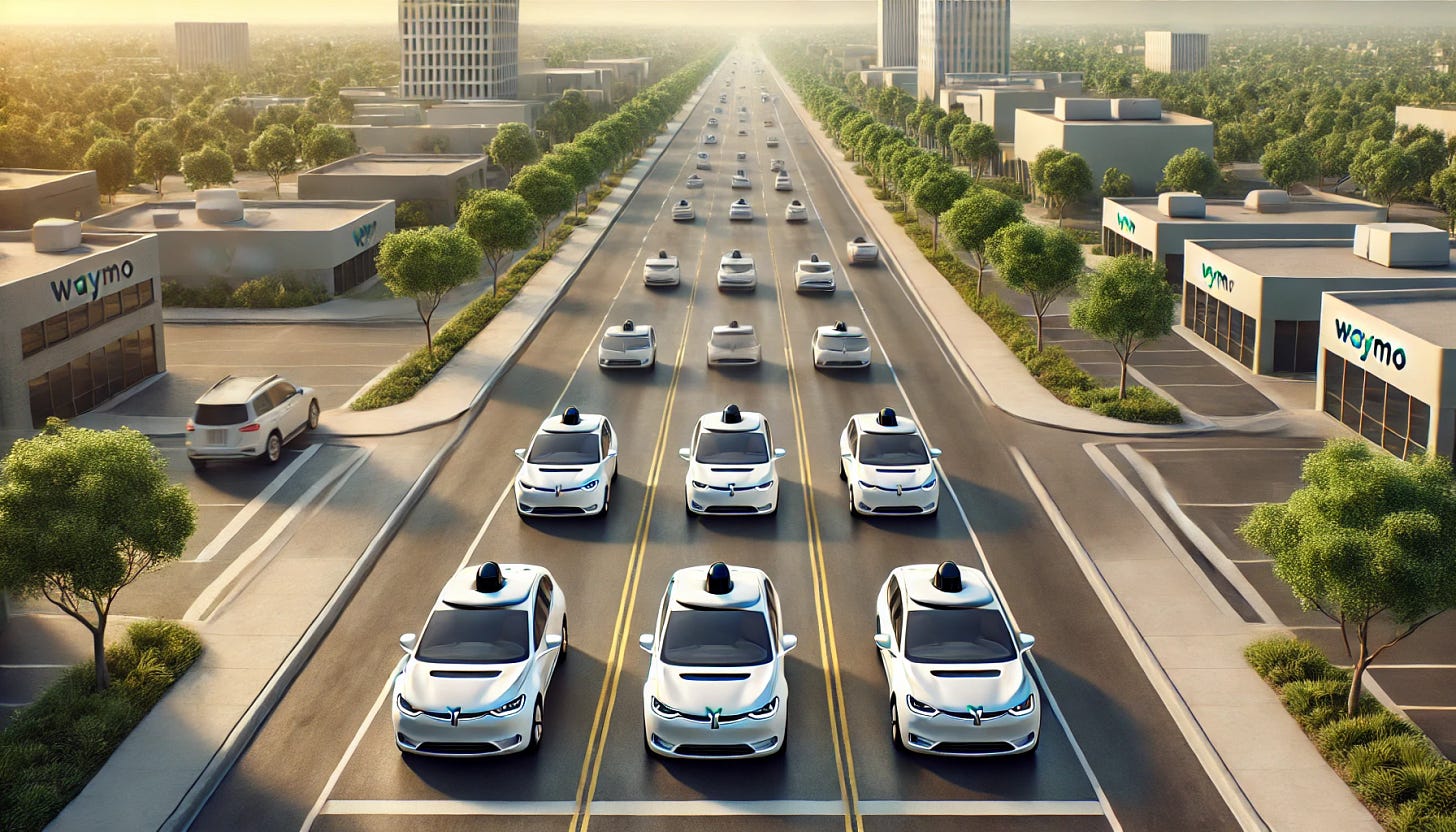
Aggressive numeric targets are a tool we should use more often. The best targets come from some sort of first-principles analysis. If you just make up a target, it might be too soft (in which case you won’t accomplish much) or too aggressive (which might push you onto an unrealistic path). A target based on mathematical analysis, even if it’s the back-of-the-envelope sort, will be more credible and more likely to land in the sweet spot that enables radical progress.
This is an AI blog, so I’m going to finish with a few ideas regarding hard targets for AI. I have complicated thoughts regarding Dario Amodei’s essay Machines of Loving Grace, but it’s an excellent catalog of promising applications for AI, such as prevention and treatment of infectious disease, slashing cancer death rates, elimination of genetic diseases, and closing gaps between the developing and developed world. Someone with the appropriate domain knowledge should propose quantitative goals in these areas. With regard to cancer, Amodei himself suggests that “reductions of 95% or more in both mortality and incidence seem possible”. An aggressive goal like this will direct energy toward a search for systematic solutions.
AI promises to upend many industries. Quantitative targets can help focus our efforts to take advantage of this. Can we set a target for increased access to health care? Or how about reduced automotive fatalities? The discussion of self-driving cars might feel different if it were attached to a goal like “saving a million lives per year9”.
Quantitative goals will also be useful for AI safety. For instance, in preparation for AI-abetted cyberattacks, we might set a goal of leveraging AI to reduce successful attacks by 90%. Again, putting a number on it (I’m not claiming that 90% is the right number) will help us to calibrate our ambitions. Can we propose quantitative goals for AI alignment, or for avoiding x risks?
What change would you most like to see in the world? Can you frame it as a number?
Thanks to Andrew Miller, Denise Melchin, Elle Griffin, and Rob L'Heureux for suggestions and feedback.
Thanks also to fellow Roots of Progress 2024 BBI Fellow Sean Fleming, whose quantitative analysis of electricity usage in Baseload is a myth triggered the idea for this post.
Current prices in the US are more in the neighborhood of ten cents, though it varies substantially by location and type of use. I believe the one cent target is for intermittent power (available only when the sun is shining) and assumes a location with reasonably favorable conditions.
Solar power has entered a feedback loop: increased demand encourages increased production, pushing manufacturers farther along the learning curve, resulting in lower prices that stimulate further demand. The demand for electricity is large enough to keep driving this cycle for quite a while yet.
Exactly how large a role clean hydrogen will play is a matter of debate. Substantial quantities of hydrogen are used today for industrial purposes (such as manufacturing fertilizer), and at an absolute minimum we will need to find clean sources for these applications. The debate is over the extent to which a net-zero-emissions economy will entail new applications for hydrogen, such as synthetic airplane fuel or seasonal energy storage.
Disclosure: I am a minor investor in Terraform.
In addition to lower energy efficiency, alkaline electrolyzers are traditionally unable to work with intermittent sources of electricity, such as solar or wind power. This is another challenge that startups like Terraform are aiming to overcome.
Note that Terraform Industries primary focus is not to sell hydrogen directly. They are also developing a cheap process for capturing CO₂ from the atmosphere and combining it with their hydrogen to make carbon-neutral natural gas.
Traditional electrolysis system designs often include multiple expensive conversion steps in the electrical path, e.g. converting solar DC to AC for transmission, then back to DC to power the electrolyzer.
A description of Chemical Mechanical Planarization, a technique for polishing a chip to be perfectly flat so that another layer of material can be deposited on top without any flaws.
About 1.35 million people per year are killed in automotive collisions worldwide. If self-driving cars could reduce this by 75% (a number I have pulled out of thin air), that would avoid about one million automotives deaths per year.
13 episoade